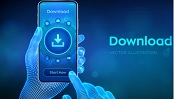
Lastly, the data is analyzed and aggregated, and the results are written back to the data lake. Data is then processed - cleansed, filtered, enriched, and tokenized if necessary. Incoming data is often referred to as raw data. Once in the data lake, you run different types of analytics - from dashboards and visualizations to big data processing, real-time analytics, and machine learning to guide better decisions.ĭata in a data lake is regularly organized or separated by its stage in the analytics process. Data LakeĪWS defines a data lake as a centralized repository that allows you to store all your structured and unstructured data at any scale. Regulations that impact how data is handled in a data lake include the Organizations Health Insurance Portability and Accountability Act (HIPAA), General Data Privacy Regulation (GDPR), Payment Card Industry Data Security Standard (PCI DSS), California Consumer Privacy Act (CCPA), and the Federal Information Security Management Act (FISMA). Given the growing volumes of incoming data and variations amongst data sources, it is increasingly complex, expensive, and time-consuming for organizations to ensure compliance with relevant laws, policies, and regulations.

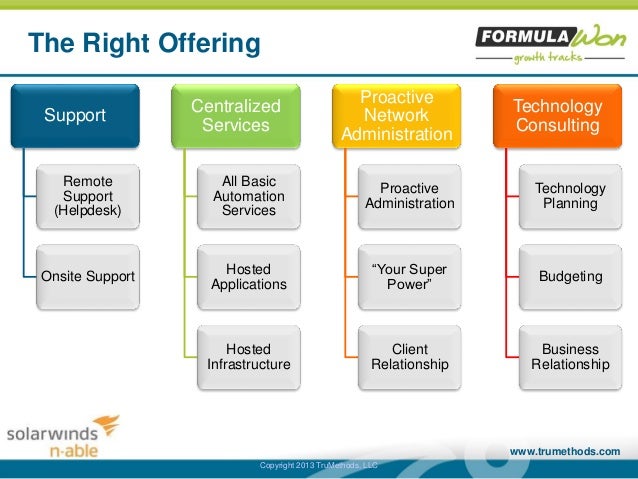
All this data is being fed into data lakes for purposes such as analytics, business intelligence, and machine learning. The volume, velocity, variety, veracity, and method of delivery vary across the data sources. External data comes from vendors, partners, public sources, and subscriptions to licensed data sources. Internal data may come from multiple teams, departments, divisions, and enterprise systems. Data typically originates from sources both internal and external to the customer. Working with Analytics customers, it is not uncommon to see data lakes with a dozen or more discrete data sources.
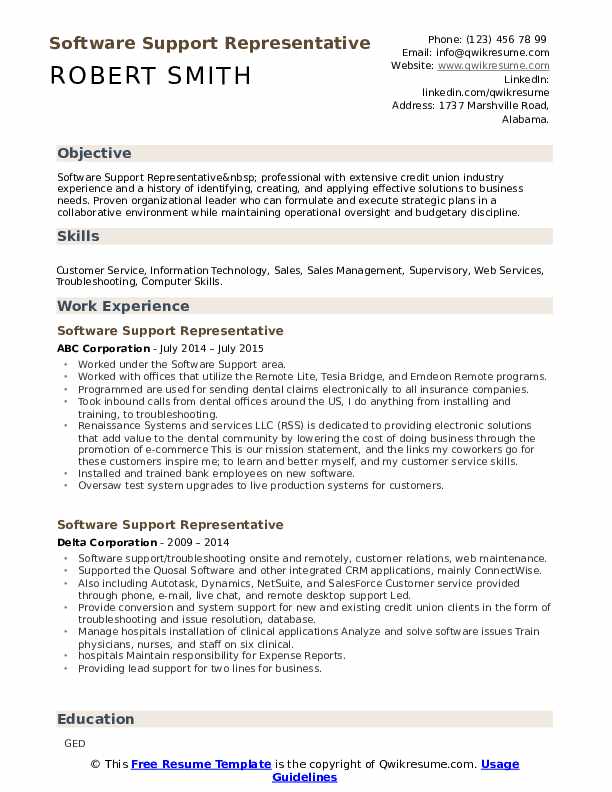
Employing Amazon Macie to discover and protect sensitive data in your Amazon S3-based data lakes Introduction
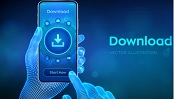